
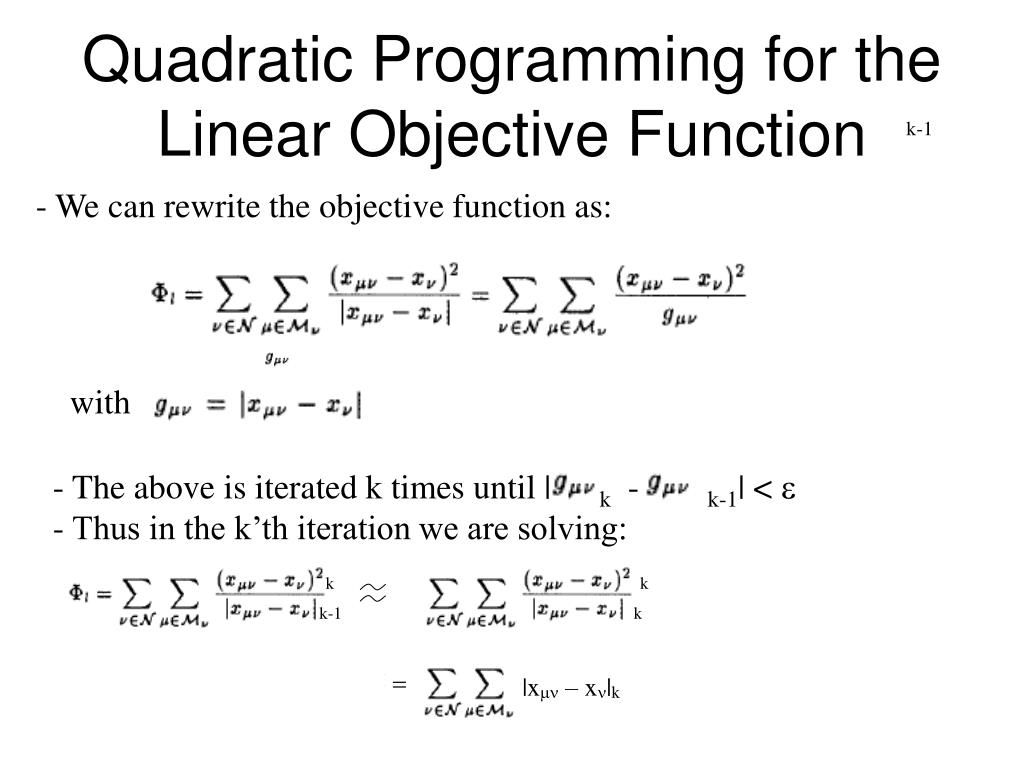
The author would like to express his sincere thanks to the anonymous referees and editors for insightful comments and useful suggestions. Nguyen Nang Tam for valuable suggestions. Nguyen Dong Yen for comments that greatly improved the paper. Questions on quadratic programming, the optimization of a quadratic objective function subject to affine constraints.

Its general form is minimize f(x) : 1 2 xTBx xTb (3.1a) over x 2 lRn subject. Such an NLP is called a Quadratic Programming (QP) problem. Supported by the Hanoi University of Industry. Chapter 3 Quadratic Programming 3.1 Constrained quadratic programming problems A special case of the NLP arises when the objective functional f is quadratic and the constraints h g are linear in x 2 lRn. Science and Technology Development (NAFOSTED) under grant number 101.01-2018.306. This research is funded by Vietnam National Foundation for As special cases, we obtain optimality conditions for the quadratic programming problems under linear constraints in Hilbert spaces. This means that if there is a solution to the primal minimization problem, then there is a solution to the dual maximization problem, and the dual maximum value is equal to the primal minimum value.In this paper, we give optimality conditions for the quadratic programming problems with constraints defined by finitely many convex quadratic constraints in Hilbert spaces. For quadratic optimization, strong duality holds if is positive semidefinite.The relationship between the factored dual vector and the unfactored dual vector is.With a factored quadratic objective, the dual problem may also be expressed as:.The Lagrangian dual problem for quadratic optimization with objective is given by: ».The dual maximizer provides information about the primal problem, including sensitivity of the minimum value to changes in the constraints.

The dual maximum value is always less than or equal to the primal minimum value, so it provides a lower bound.
